Audio Transformer
Groupie
- Messages
- 37
Just over a year ago, after NAM started gaining massive traction, I suggested to Steve Atkinson that we should address a core issue with real-time inference in NAM models: aliasing.
As we all know by now, neural networks are black boxes, and trying to fix aliasing through oversampling isn’t (yet) possible.
I proposed to Steve that we specifically train NAM models to learn how to correct aliasing. However, he told me that NAM was already state-of-the-art and that aliasing wasn't really a concern — most users didn’t even notice it.
Still, as an AxeFX3 user, I had asked Cliff to look into integrating NAM into the Fractal ecosystem, given its incredible fidelity and null tests that outperformed everything else out there, including Tonex and NDSP captures.
But Cliff quickly pointed out the aliasing issue and concluded that neural networks couldn’t truly eliminate aliasing artifacts.
That’s when, in February 2025, I had an idea: what if we trained a NAM model using signals that exposed aliasing the most clearly? Maybe that would force Wavenet to "fix" it to match the clean reference.
I started training a NAM model using only sine sweeps between 20kHz and 24kHz (NAM runs at 48kHz) — and BAM! It worked!
The model massively reduced aliasing — although the sound was quite different from the real amp: softer and fuzzier. But it worked.
Then I thought: what if I combined Steve’s WAV3 training set with the high-frequency sine sweeps?
That worked even better — very low aliasing and a tone faithful to the real amp.
But after more testing, I noticed that if the input volume during inference was very different from the one used for training (with the sweeps), the anti-aliasing effect weakened.
So I decided to create sine sweeps at lots of different levels to help the model generalize to any volume.
I also started using a combination of ascending and descending sweeps, both linear and logarithmic — an idea Marcelo had used in his TTS experiments.
That turned out to be brilliant.
Eventually, with Andrei, Marcelo, and others, we basically started a friendly competition, trying to create the best "super input" — something that could simultaneously improve NAM fidelity (better null tests even outside of the training distribution) and crush aliasing, pushing it down to nearly -80dB!
In the end, though, I got kicked out of the NAM Facebook group for pushing a bit too hard to get the super inputs and Andrei’s XStd architecture integrated into the main project — even though I totally get that their goal is to keep NAM stable and standardized.
As Steve said: it’s a free and open-source project.
Since then, I’ve been running a ton of experiments to make sure the new models aren’t just "cheating" by being good only at sine sweep tests.
I made the tests increasingly complex and rich, and the results were great: the models generalize beautifully for complex, musical signals — well beyond what’s detectable.
Also, it’s super exciting to see Slammin Mofo release free packs using Marcelo’s super inputs — they work fantastically and produce the kind of killer graphs I'm sharing here along with the download link.
As we all know by now, neural networks are black boxes, and trying to fix aliasing through oversampling isn’t (yet) possible.
I proposed to Steve that we specifically train NAM models to learn how to correct aliasing. However, he told me that NAM was already state-of-the-art and that aliasing wasn't really a concern — most users didn’t even notice it.
Still, as an AxeFX3 user, I had asked Cliff to look into integrating NAM into the Fractal ecosystem, given its incredible fidelity and null tests that outperformed everything else out there, including Tonex and NDSP captures.
But Cliff quickly pointed out the aliasing issue and concluded that neural networks couldn’t truly eliminate aliasing artifacts.
That’s when, in February 2025, I had an idea: what if we trained a NAM model using signals that exposed aliasing the most clearly? Maybe that would force Wavenet to "fix" it to match the clean reference.
I started training a NAM model using only sine sweeps between 20kHz and 24kHz (NAM runs at 48kHz) — and BAM! It worked!
The model massively reduced aliasing — although the sound was quite different from the real amp: softer and fuzzier. But it worked.
Then I thought: what if I combined Steve’s WAV3 training set with the high-frequency sine sweeps?
That worked even better — very low aliasing and a tone faithful to the real amp.
But after more testing, I noticed that if the input volume during inference was very different from the one used for training (with the sweeps), the anti-aliasing effect weakened.
So I decided to create sine sweeps at lots of different levels to help the model generalize to any volume.
I also started using a combination of ascending and descending sweeps, both linear and logarithmic — an idea Marcelo had used in his TTS experiments.
That turned out to be brilliant.
Eventually, with Andrei, Marcelo, and others, we basically started a friendly competition, trying to create the best "super input" — something that could simultaneously improve NAM fidelity (better null tests even outside of the training distribution) and crush aliasing, pushing it down to nearly -80dB!
In the end, though, I got kicked out of the NAM Facebook group for pushing a bit too hard to get the super inputs and Andrei’s XStd architecture integrated into the main project — even though I totally get that their goal is to keep NAM stable and standardized.
As Steve said: it’s a free and open-source project.
Since then, I’ve been running a ton of experiments to make sure the new models aren’t just "cheating" by being good only at sine sweep tests.
I made the tests increasingly complex and rich, and the results were great: the models generalize beautifully for complex, musical signals — well beyond what’s detectable.
Also, it’s super exciting to see Slammin Mofo release free packs using Marcelo’s super inputs — they work fantastically and produce the kind of killer graphs I'm sharing here along with the download link.
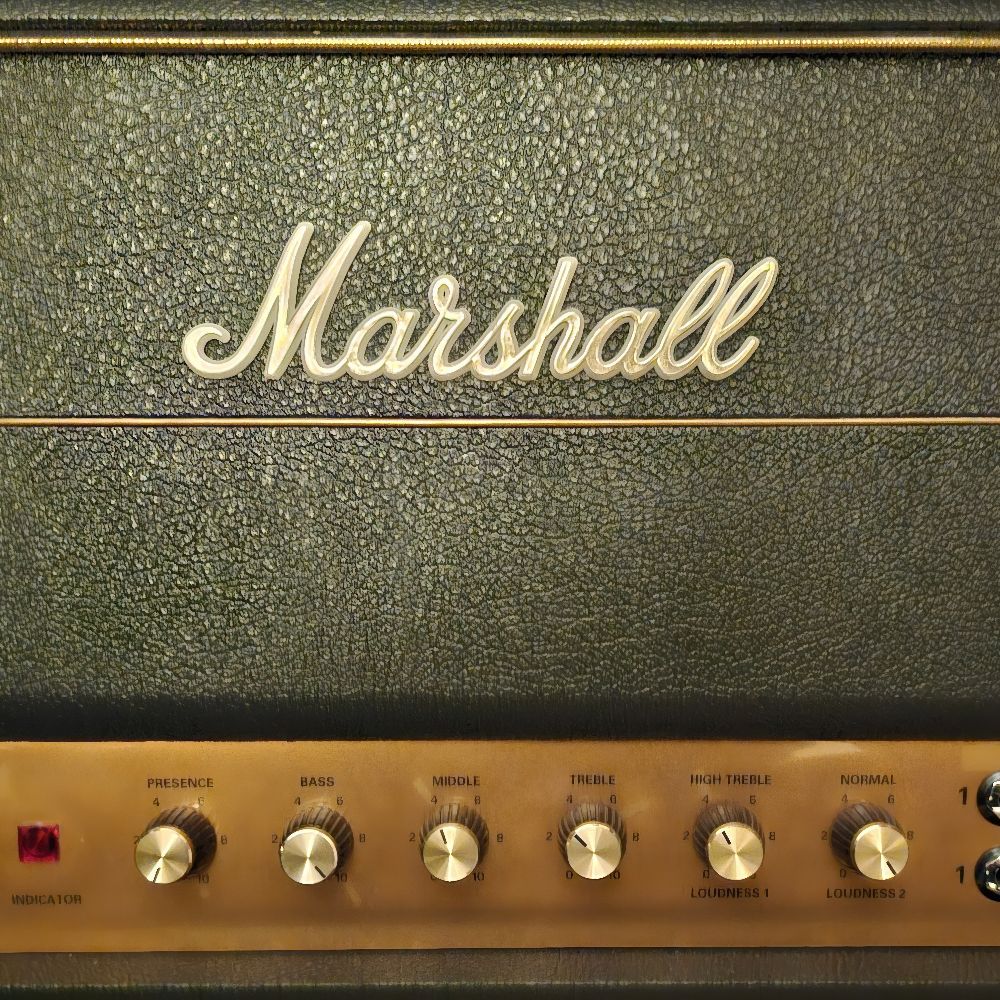
Marshall 1959 BRBS SIR #34 Experiment NAM Profile · TONE3000
Check out slamminmofo's neural amp head capture for guitar, bass and recording: This capture set serves as an expirment. All the captures are based on the same amp tone generated by a Marshall 1959 BRBS #34 SIR mod. The only differences are the employed test signals and the training...
www.tone3000.com